AI in healthcare: the impact on decision-making
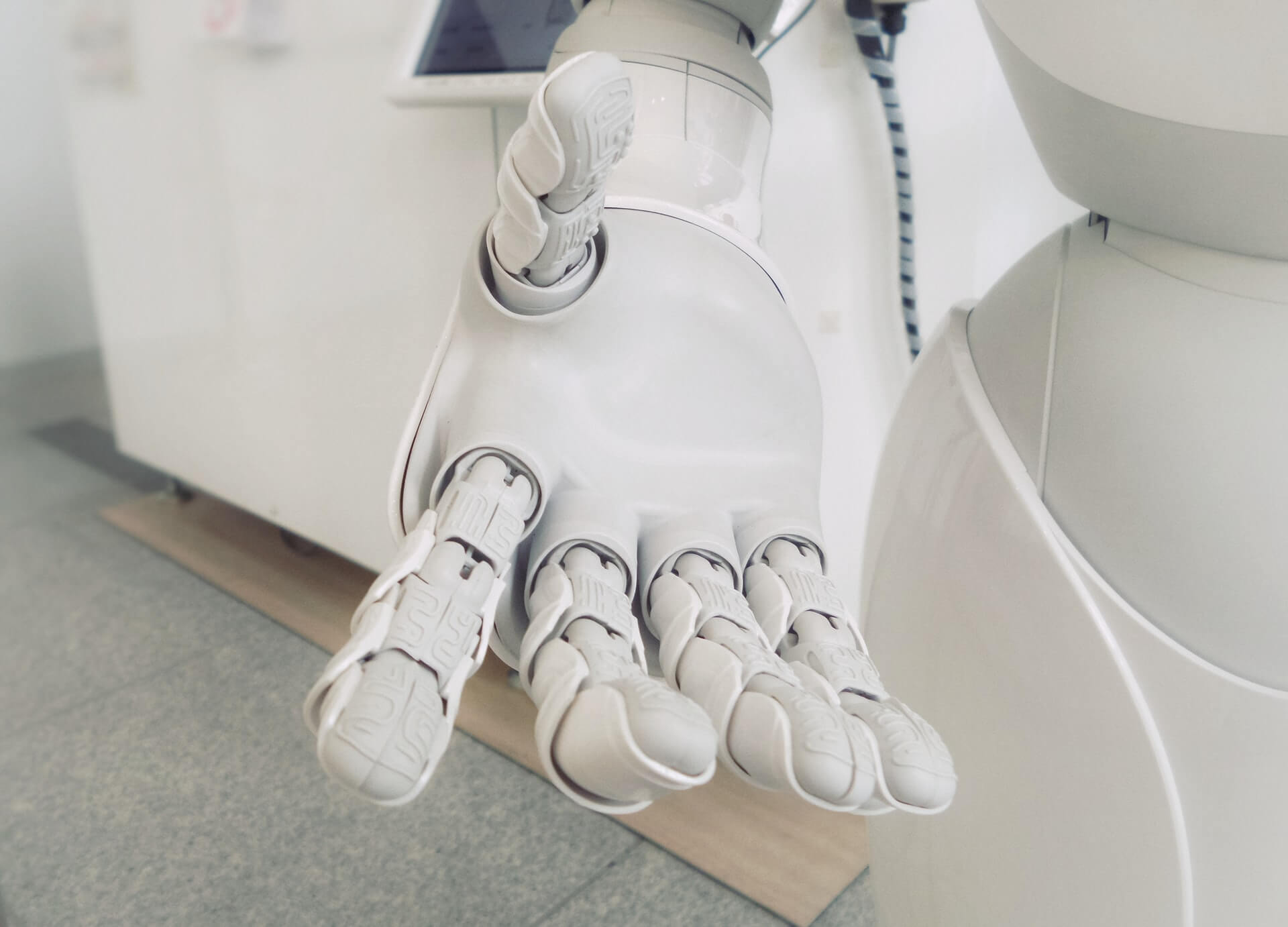
Despite controversial views surrounding the adoption of AI into clinical practice, in many cases, AI in decision-making is not just an option but a necessity.
Decision-making is an integral and crucial cornerstone of any business, directly affecting the course of affairs. If a wrong solution can expose some businesses to financial losses, decision-making in healthcare has no margin for error. Why? The answer is obvious: human life is at stake.
Healthcare is the convergence of scientific data and personal judgment, while modern healthcare also embraces technology. It is precisely tech advancement that links these two elements, bringing them closer and closer every year. The result is AI-enabled decision-making as a vital component in diagnosis, treatment, analytics, care delivery, and critical medical outcomes.
Medical organizations can bypass AI-enabled decision-making by relying solely on the specialists’ expertise, but will it be the right decision? Despite contradictory opinions among healthcare-related people regarding the use of AI in medicine, an innovative approach can also yield positive results.
Decision-making in healthcare brings its own challenges
Healthcare decision-making is complicated due to the nature of collected data, new options, and pressure that increases every day. Besides, factors such as time constraints, teamwork, human factors, and case complexity [1], cause medical decision-making to be even more intricate and time-consuming. The interplay between these factors at different levels of the decision-making process can only increase the complexity of problems.
Given the vast amount of information and current pace of healthcare advancements, med professionals face a major obstacle in their medical education journey: time shortage.
Medicine does not put up with downtime, while decision-making requires no hurry. Therefore, a poorly technically-equipped medical company is more than likely to lose to its tech-savvy competitor, which has chosen the efficient "more quality in less time” strategy. In turn, artificial intelligence comes to the rescue, supporting the process of decision-making by HCPs rather than making the decisions for them.
Mistakes are bound to happen
According to a survey conducted by the Institute for Healthcare Improvement, misdiagnosis is considered the main medical mistake [2].
Meanwhile, according to the same survey, the main causes of mistakes are lack of attention to detail. After all, the human factor should not be overlooked. As in any business, mistakes cannot be avoided, even in healthcare. However, AI is capable of reducing them. Nevertheless, there is a justification for medical mistakes.
Firstly, we are witnessing a rapid pace of healthcare advancements, which means more and more data to be obtained by medical-related people under time pressure.
Secondly, the COVID-19 pandemic has shown the vulnerability of the world to new viruses, triggering a rapid virus control process and speeding up decision-making.
Thirdly, the pandemic is considered the main propeller of patient growth on a global scale, putting pressure on medical personnel.
Finally, physicians' lack of confidence in AI reduces the chance of adapting it as a tool to reduce errors in decision-making. Trust can be affected by some human factors, such as experience, user biases and attitudes toward automation, and the reliability of the technology itself. But the fact is that any innovation undergoes an “introductory and approval” period. It is more important to find out how to apply it correctly in order to achieve better outcomes.
AI in medical decision-making: the impact is significant
According to Grand New Research, the global market for artificial intelligence in healthcare was estimated to be $10.4 billion in 2021. It is expected to grow at a compound annual growth rate of 38.4% from 2022 to 2030 [3].
Indeed, AI is gradually gaining its place in many areas of medicine. Given the favorable long-term prospects for machine learning models usage, fully AI-adapted medicine with digital product development at its core is the not-too-distant future rather than a fantasy. In addition to AI-assisted clinical decision support, population health management, care delivery, wellness, and more areas are evolving with digital technology as the foundation for a rapid tech shift.
Clinical decision support systems as a current approach in healthcare decision-making assistance
Medicine has already made a quantum technical leap forward by introducing AI algorithms-focused software platforms into daily practice. These AI algorithms are set to filter, organize and search for patterns in large data sets. Ultimately, the system provides a likelihood-based analysis from which clinicians can jump to conclusions. Given that some jurisdictions do not currently consider the algorithms as final decision-making tools, AI systems are mostly regarded as assistants in making final clinical decisions.
Besides, the growing hardware computational power provokes the introduction of systems that connect electronic medical records with other data sources: research databases, labs, pharma systems, and even IoT data. Subsequently, a single data hub is used by AI-based clinical decision support systems (CDSSs).
AI/ML-enabled CDSSs are capable of analyzing large amounts of data, suggesting next steps for treatment, making predictions, and at the same time facilitating the workflow. For example, the system can simplify patient-specific dosing decisions after a physician has entered the necessary metrics. Using the existing data that includes RWD and RWE and data entered by the physician regarding a particular patient, the algorithm will make recommendations on the appropriate dosage for the patient's treatment. Besides, the use of such systems reduces clinical errors.
Areas where AI can act as the right hand in decision-making
The use of artificial intelligence and advanced analytics facilitates making quick and accurate decisions. For example, a digital copy of the patient, i.e., a patient duplicate created with advanced technologies, will help reach error-free insights, predict the results of procedures, and ensure the right choice of treatment and therapy. What are the areas where AI-supported decision-making has a significant impact?
1. Diagnosis
AI-enabled systems can streamline the diagnosis procedure for healthcare providers by analyzing collected data on previous medical records and cases. Symptoms, clinical diagnosis data, family history, and demographic data are just a few examples of such data. The system also uses a "questionnaire" approach, asking patients after symptoms have been entered. Thus, the chances of a successful diagnosis will increase many times over.
2. Treatment
AI technologies can also be effective in the subsequent stage — treatment prescription. After collecting the necessary data, a system can draw accurate conclusions about the patient's needs and provide a physician with a possible scenario of treatment and care pathway.
3. Disease prediction
By 2030, the number of people over 60 is expected to increase by about 56% [4]. Besides demonstrating the success of modern medicine, such data also highlights certain challenges since older people tend to have more complicated healthcare needs.
Nevertheless, AI can help predict illnesses, thereby allowing for early treatment or even disease prevention. For example, vast amounts of data on chronic diseases can be more thoroughly vetted so that diseases can be diagnosed and treated at an earlier stage.
In addition to providing information on patient care, AI can deliver valuable insights about your healthcare organization's performance. Thus, tech solutions can help assess commercial business performance, determine patient satisfaction levels, and predict future insights based on analyzed data. In this way, you can both assess business performance and understand more customers’ pain points that need to be solved.
4. Risk assessment
CDSSs can function as one of the methods for estimating treatment risk and success. For example, the system allows in-depth data processing in a short period of time to suggest diagnoses, risk factors, and patient-specific outcomes. This provides a more personalized approach with a more likely positive outcome.
It is also worth mentioning that AI is often regarded as an opportunity rather than a necessity.
AI is a necessity:
- When the lack of decision is the worst decision, and the scale makes it impossible to perform the decision-making for everyone.
- In the case of high-throughput screening (narrowing down the possible candidates to a smaller subset).
- When the data is so high dimensional that there is no comprehensive way to understand it in a clear manner.
AI is an opportunity:
- In the case of a large amount of data on a particular theme — there is a risk that someone could miss important information and make the wrong decision.
- For the discovery of new treatment and diagnostic methods.
Although AI brings several benefits, the intricacy of its implementation in a clinical setting becomes one of the main obstacles for medical companies. What else prevents companies from switching to AI-infused medicine?
Key hurdles to AI adoption hindering informed decision-making
- Tech issue
According to the survey [5], 56% of respondents believe that the low maturity of the external ecosystem impedes the acceleration of AI initiatives. This also includes low levels of digitalization, insufficient training information, and a lack of a single data warehouse.
- ROI issue
53% of respondents consider the inability to quantify the benefits of AI to be one of the main obstacles.
- Trust issue
47% of the respondents explain the slowdown in AI integration by the medical personnel's mistrust of "machines".
- Expert issue
40% of business leaders state that the key bottleneck for them is the lack of talent able to design, implement, scale and support AI systems.
We agree that adapting AI within medicine is often a tough and resource-intensive “ordeal” that many businesses give up on. But behind every challenge and the crucial decision that requires immediate action comes the company's strength and growth.
To pass this endeavor with dignity, an experienced team of AI experts is hard to do without. As Brandmed, we rely on tech AI specialists with in-depth knowledge of the business, market, and, of course, medicine. By combining all this, we can help you bring your AI project to life by taking the path of least resistance. How about a discussion?
References:
- National library of medicine/ Alicia M Zavala, Gary E Day, David Plummer, Anita Bamford-Wade: Decision-making under pressure: medical errors in uncertain and dynamic environments, https://pubmed.ncbi.nlm.nih.gov/28578757/, [last accessed: 21.04.2022].
- The Institute for Healthcare Improvement, Americans’ Experiences with Medical Errors and Views on Patient Safety, 2017, http://www.ihi.org/about/news/Documents/IHI_NPSF_Patient_Safety_Survey_Fact_Sheets_2017.pdf, [last accessed: 21.04.2022].
- Grand view research, Artificial Intelligence In Healthcare Market, 2022, https://www.grandviewresearch.com/industry-analysis/artificial-intelligence-ai-healthcare-market, [last accessed: 21.04.2022].
- World population aging report, 2015, https://www.un.org/en/development/desa/population/publications/pdf/ageing/WPA2015_Report.pdf, [last accessed: 21.04.2022].
- Impediments to AI adoption: What enterprises “perceive” vs. what they actually “experience”, https://www.businessprocessincubator.com/content/impediments-to-ai-adoption-what-enterprises-perceive-vs-what-they-actually-experience/ , [last accessed: 21.04.2022].